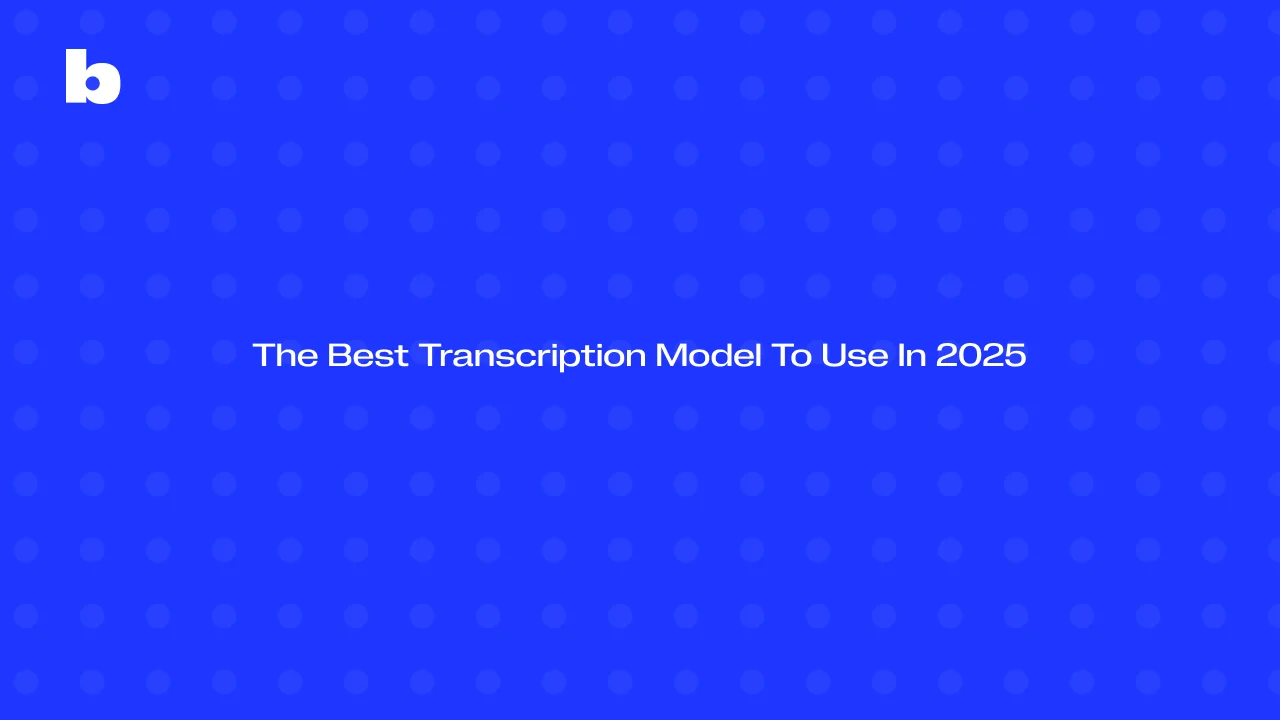
When choosing the best model of transcription for you, it’s important to find one that offers high accuracy, speed, and even flexibility. The right model can tackle challenges like varying accents, background noise, language identification and different speech patterns, making it ideal for a variety of tasks such as transcribing meetings or supporting multiple languages.
As you read you'll discover which features you need to prioritize, how transcription models work, and the benefits that they can bring to enhance your productivity, communication, and accessibility in your work or personal projects.
What Are Speech-To-Text Models?
Speech-to-text models are tools that turn spoken words into written text. Using advanced speech recognition, they use audio and create clear, accurate transcripts. These models are trained on all kinds of audio, so they are great at handling different actions, languages, and even noises in the background, making them super reliable.
What makes it so useful is the ability to adapt. They can recognize the context of what’s being said and even figure out when people are switching languages in the same recording. Whether it's a messy meeting recording or a polished podcast version, these tools work hard to give you solid results. They are perfect for transcribing interviews, captioning meeting notes, and logs and summaries.
People and businesses use these models in all sorts of ways. For example, developers add them to apps to handle transcripts of voice commands, while teams rely on them to keep track of meetings or achieve important conversations. They are not just about making life easier – they’re about saving time and cutting out the boarding stuff, like manual note-taking.
With how far technology has come, these models can now do even more; they can process audio in real-time, recognize different languages, and work with all kinds of files. Plus, they are available in different different versions, so there’s usually something that fits your needs.
The language model: bringing meaning to the transcription
The language model is what makes a transcription feel natural and meaningful. It takes all data and provides context to figure out how the words should fit together. Instead of just translating sound into text, it makes sure that the transcription flows and makes sense. For instance, it knows which words are likely to follow each other and can spot it is based on how people talk.
This is the best model for training on huge, diverse datasets. Language models have gotten good at this, helping create transcriptions with exceptional accuracy that feel more like real conversations when you engage with them.
The acoustic model: converting sounds to letters
The acoustic model is where the magic of turning sound into text begins. It listens to the data, breaks it down into patterns, and matches those sounds to the right letters and words. Even when there’s noises or unclear speech, the model can figure out what is being said.
By training on a wide variety of voices and accents in diverse datasets, it learns to handle just about any kind of audio input that you provide it with. This is why it can transcribe spoken words with impressive accuracy, making it a critical part of any speech-to-text system.
Best Speech-To-Text Open Source Models
If you’re looking to dive into speech-to-text technology, there are some excellent open source models out there. These models are known for being flexible, accurate, and able to handle a wide variety of languages across the board.
Whether you’re working on a personal project or building something for a business, these models a great options for integrating speech recognition into your apps. Years a tiny look at some of the best open source models available today, each with its unique strengths.
Whisper
Whisper is an open-source speech recognition system that was crafted by OpenAI. It’s trained on a huge collection of others from the web – and estimated 680,000 hours worth. This training helps it to transcribe speech in English and other languages, and it can even translate speech from English languages into English, making it useful for many different language needs.

Whisper works using a model that breaks down the audio into 30-second chunks and turns them into something called log–Mel spectrograms. These spectrograms are processed by a system that then predicts the output text. It’s not just about turning sound into words, though – Whisper can also do things like identification, at times stamp, and handling multilingual transcription all within the same process.
Whisper stands out because of its exceptional accuracy alone. It can handle different accents, deal with noises in the background, and understand technical terms because of the broader range of data it’s been trained on.
DeepSpeech
DeepSpeech is an open-source recognition of speech tool created by Mozilla in 2017, based on the DeepSpeech algorithm from Baidu. It works by converting audio into text using a deep neural network, and a language model that helps to improve the accuracy and the flow of the transcription. The system was trained on different data, so it works as both a transcriber and a grammar checker. DeepSpeech's evolution can be used for training and real-time tasks and supports multiple languages and platforms. It’s also flexible and can be retained to suit different needs.
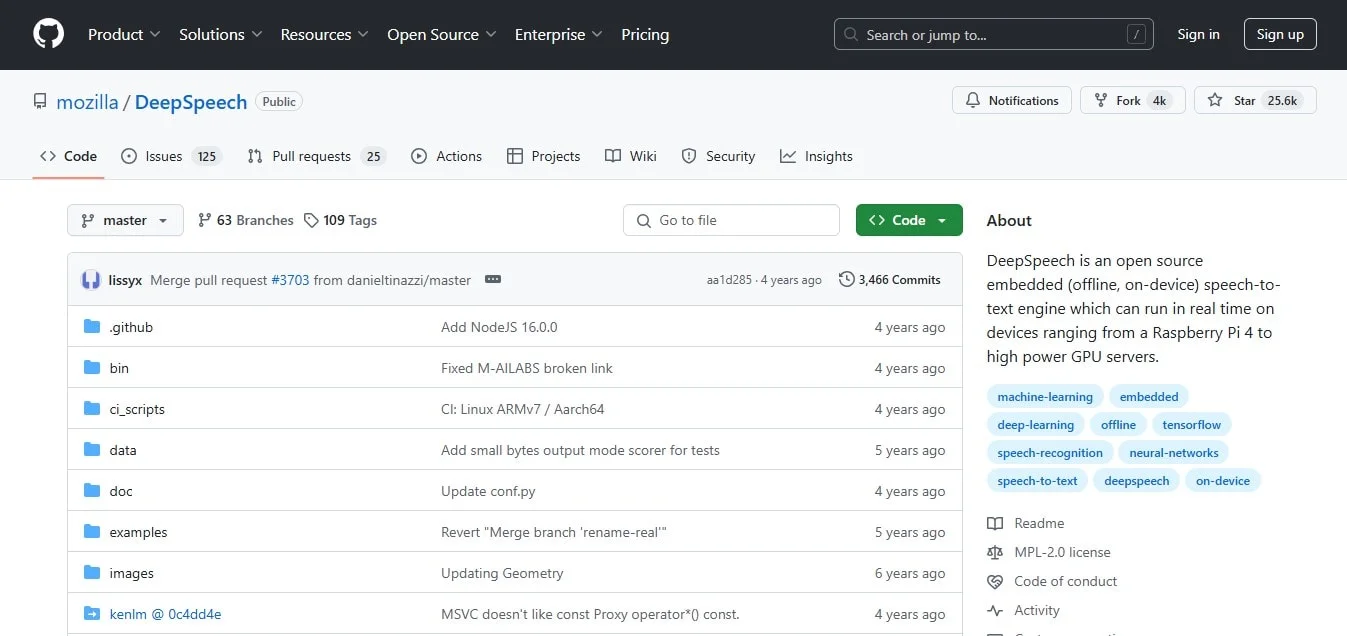
With that being said, the limitations when compared to more advanced systems like Whisper. For instance, DeepSpeech can record audio for up to 10 seconds, so it’s more useful for short tasks like command processing, but not really for longer transcriptions.
Also, the corpus is fairly small – around 14 words and 100 characters per sentence. To make training for foster, developers often need to split up sentences or remove common words. While there are plans to extend audio recording, it still won’t match the performance and accuracy of more modern models.
Kaldi
Kaldi is a tool kit for doing speech to text recognition, it was designed to be flexible and easy to adapt. It’s both with a modular approach, making it easier for developers to customize and extend. This means that Kaldi isn’t just for speech-to-text systems – its algorithms can be reused for a variety of other AI applications, giving it a lot of versatility.

Unlike reading speech recognition systems, Kaldi is more of a framework for building your own. It works with common audio datasets, to create ASR programs that can run on regular computers, android devices, or even in web browsers using web assembly. While browser systems still have some limits, they are an exciting step towards fully cross-platform speech to take solutions that don’t need server-side processing.
SpeechBrain
SpeechBrain is a versatile tool kit that was designed to handle everything related to conversational AI. It can manage tasks like speech-to-text translation, speech synthesis, and working with large language models, making it a goal tool for crafting natural interactions with chatbots or voice-based systems.

One of the best things about SpeechBrain is its academic roots. It was developed with help from over 30 universities around the world and has a big, active community. This community has more than 200 training guides using 40 different datasets, covering many tasks like speech and text processing.
Wav2vec
Wav2Vec, developed by Meta, is a speech recognition tool designed to work with unlabeled audio data. It is to make ASR (automatic speech recognition) available for more languages, including those that don’t have access to a lot of labeled datasets for training.
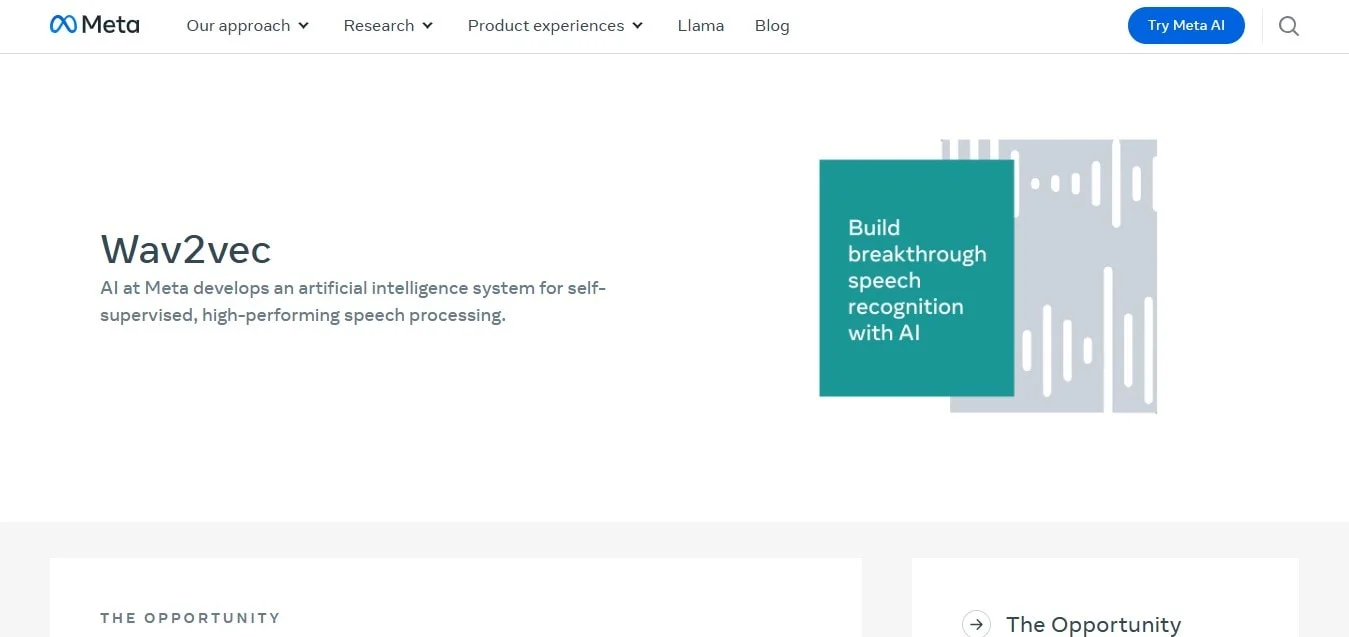
The big idea behind this is to tackle a major limitation of traditional ASR systems: they need a huge amount of speech audio paired with written transcriptions, which is impossible for many of the world's languages and dialects. Wav2Vec solves this by using a self-supervised learning approach. Instead of relying on labeled data, it learns by predicting tiny mosque audio segments as if there were tokens, kind of like how language models predict missing words.

Conclusion
Choosing the right transcription tool or app that can transcribe audio can make a huge difference in how well you capture your notes and important conversations. Bluedot is an excellent choice for recording and transcribing meetings, especially when screen sharing becomes involved. It’s not just about transcription – Bluedot offers so much more.

It helps you craft meeting templates, automatically generates emails after your meetings, has AI note-taking tools, and offers call transcription software. With Bluedot's new AI chat feature, you can now interact with and control everything more naturally.
Bluedot is designed to make your meetings more organized and efficient, making sure that you never miss out on key details. Because Bluedot doesn't have a bot that joins your meeting, it's best to learn what the best practice is to obtain consent to record meetings.